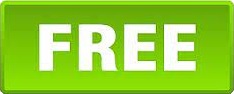
.jpg)
In the following section, we will present our CNN model in detail. Brief descriptions of time domain and spectrogram feature of HRRP are introduced in Section 2. The remainder of this article is organized as follows.

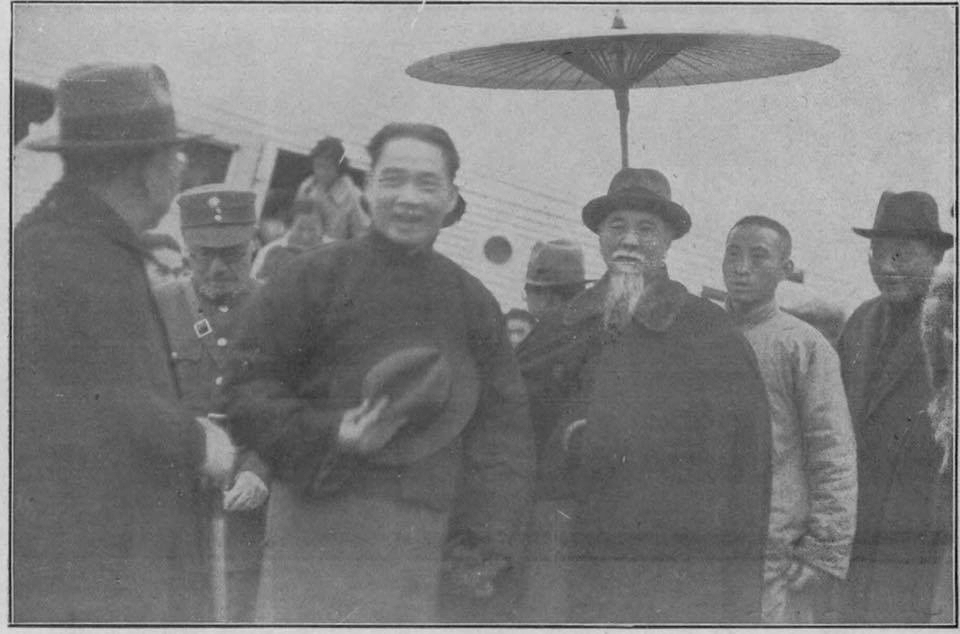
By measuring the reconstruction error between the input and the output of the decoder, the model can identify whether a sample is an outlier or not. Specifically, the proposed approach uses a CNN as encoder and a deconvolutional neural network as decoder. Therefore, we plug a decoder into the model to realize the outlier rejection. Moreover, it is unreasonable to recognize a target that does not belong to any class of the existing template database. Since most of the targets are uncooperative or even hostile in real-world RATR task, it is not practical to acquire complete training database. Hence, targeting the properties of spectrogram, a novel CNN model is proposed for HRRP ATR, which, different from the conventional 2-D CNN for images, considers the spectral vector at each discrete time (range cell) as a whole and realizes the convolution operation along time dimension. By contrast, the spectrogram feature is a two-dimensional (time and frequency) representation of a HRRP signal, which embeds the frequency domain property of the target and reflects more phase information. Nevertheless, the time domain only characterizes the amplitude of the target signal which provides limited information for feature learning. In the 1-D CNN, the convolution operation only takes place at range dimension. In this paper, we first develop a one-dimensional CNN recognition procedure for the time domain HRRP, which represents the amplitude of projection of the target scattering centers onto the radar LOS and is widely used in RATR. They have been successfully applied to kinds of recognition tasks and exhibited better performance than fully connected networks. Furthermore, the convolutional layer alternates with the pooling layer to learn the features, which leads to the local invariance of translation, scaling, and shift. Differently, convolutional neural networks (CNNs ) explicitly exploit structural locality in the feature space and can extract more descriptive features that exist in signals. However, these two models are based on fully connected nets and may not capture the structural information among the range cells of HRRP layer by layer, since HRRP reflects the distribution of scatterers in target along the range dimension.
.jpg)
employ the average profile as the correction terms and stack a series of Corrective Autoencoders to extract features from HRRP. employ stacked denoising autoencoder (SDAE ) to learn the robust representation of the original HRRP. In fact, several deep learning models have been developed for HRRP-based RATR. Recently, non-linear, deep networks have been successfully employed in various real-world tasks thanks to their powerful expressive ability. However, all those methods build linear and shallow architectures that limit their capability to represent the complicated HRRP data. and Zhou apply sparse constraint on the feature vectors and solve the problems via l 0-minimization. Considering the sparsity within the HRRP signals, Feng et al. In, the researchers employ factor analysis (FA) model to project and recognize HRRPs in a low-dimensional latent feature space. In, the principal components analysis (PCA)-based feature subspace is constructed to minimize reconstruction error for RATR. Generally, such sophisticated features perform well in practice but they heavily rely on personal experiences and prior knowledge.īesides those hand-crafted feature methods, data-driven RATR approaches have attracted increasing attention in past years due to their ability to learn useful features from the dataset automatically. In, the researchers investigate the recognition methods based on spectra features, which perform the recognition task in the learned feature subspace via specific physical meaning. In, RELAX-based algorithms are employed to extract the location information of predominant scatterers from HRRP data as features for recognition tasks. Many researchers explore various feature (representation) learning methods for HRRP-based RATR.
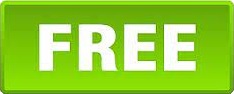